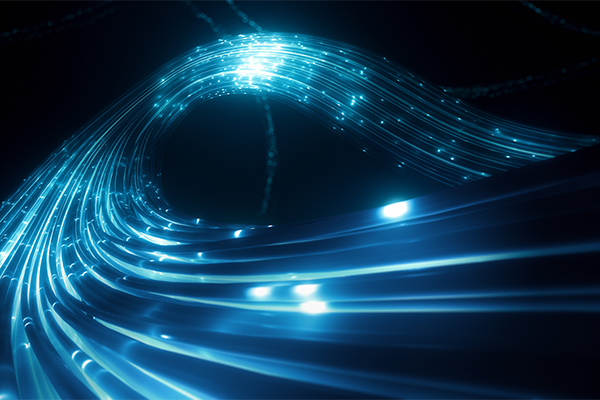
When I graduated from Uni in the early 1990s I had specialized in artificial intelligence and machine learning. If I had graduated 10 years later I might have been what you now call a data scientist but in 1990s, Google didn’t exist as a corporate entity and no one wanted data scientists. So I had to content myself with retraining into content and identity management and that in time led to me to the noble pursuits of personalization and data driven marketing. I have fortunately maintained my love of data and data modeling and I’d like to share a few insights on big data, data analytics and what I’d like to call new world data analytics.
Old world data analytics – Extract, Transform & Load
Old world analytics is the structure of people, process & technology surrounding the Extract, Transform & Load (ETL), Data Warehousing (DWH) and Business Intelligence (BI) used by most enterprises today. Old world analytics is slow moving, potentially suffering from political subversion or exclusion of input data by silo’d departments and experiencing hours, days or weeks of latency. Insights, trends & analysis derived from old world analytics also tends towards lag indicators of business decisions and can fail to provide meaningful insight into future business direction.
Big data is often positioned as a way to rectify the latency and lag-indicator problems of old world analytics with special focus being placed on the hidden or latent value data not currently being brought to bear and the ability to process and a wealth of external & new data sources along side existing enterprise data sources.
The truth is that technologies associated with big data are distributed batch processes based around map-reduce solutions so they are just as prone to subversion and further latency and have more in common with old world analytics models and further isolate business leaders from real time insights available in enterprise data.
The 3 V’s of big data – approach with care
When talking about big data the 3 V’s, Volume, Variety, Velocity, are often paraded as the best description. The 3 V’s were first coined by Gartner in 2001 and honestly, there firstly isn’t really anything new about these terms or their constraints on data management capacity and secondly, they are a really good way to sell you lots of storage, computational power, advanced data analysis and data modeling tools & specialist data scientists. But at the end of it all, what Value do you see?
The problem with the 3 V’s is that they are set up to look for “needles in the hay stack” when in reality we are far more interested in accurately modeling the giant logs lying across our respective hay stacks that we already understand well as analysts and business leaders. The big logs are the models that drive our businesses and big data is merely the latest name for a range of sold products that try to improve our understanding of these models.
The 3 C’s of big data and 6 questions – more useful guidelines
Instead of the 3 V’s I start with the 3 C’s or Complexities of Big Data instead:
- Context – what are you collecting all this data for? What business problem are you solving?
- Comprehension – do you know what all you data means? Is it all properly tagged and able to be associated with other data sets?
- Collaboration – how can anyone else play with this without costing $mm and can we easily associate data from other sources?
Instead of the 3 Vs, I ask executives to consider these 6 questions:
- What industries or parts of your business make the most money?
- What models drive those industries/businesses? Do any models drive more than one industry/business? How much money are those models worth?
- What data do you need to build those models? Do you need raw data or rolled up data? Do you need PII data or will anonymous segments do?
- Who has the data you need to build those models? Will they allow you to use it?
- How can you collaborate to get the data/segments? How much is it worth to you to get that data? What will you pay? How much will you make?
- FINALLY… what tools/processes/skills do you need to put in place to execute on the data?
You may find that you don’t need Hadoop, or even data scientists – you don’t want to spend a lot of time & resources on hardware, consultants & technology and lose years of business advantage working that out – and you certainly don’t want a more senior business leader coming around and saying, “what’s all this kit for and who authorized it?” if you don’t have clear business driven answers for why.
Approach big data from a business first perspective
Instead, approach big data from a business first approach and don’t let technology drive your business agenda. You will most likely discover that what you really need is a way for business leaders to collaborate on disparate data sets with more focused application of data experts; you will want the ability to build and iterate on ad hoc queries quickly and you will want the ability to action your data insights and feedback success scores quickly to drive the success of your business.
New world data analytics and fast data
What is far more valuable than big data is: fast data, accessible data, actionable data & measurable data. This isn’t just about the speed or accuracy of insights delivered. It is also about the accessibility of the insights to business leaders. You don’t want to have to engage in a 6-12 week big data technology discovery & delivery cycle to ask each data driven question and you don’t want to wait days or weeks to get your data insights to market and months more to find out if your data driven initiatives are working. You also don’t want the scalability of your business to be determined to the number of data scientists and technical analysts you can hire. The right solution for new world data analytics needs highly scalable people, process and technology solutions. It needs a strong core of technology & analysts but those elements cannot be allowed to become a bottleneck. New world data analytics needs to be fast, accessible, actionable & measurable.
New world data analytics first and foremost is a shift in thinking about how we manage and who can access data. Instead of batching data into an ingestion process we first stream it directly into a real time pipe. Into that pipe we also read all other data sources such as device data, social data, site & app data and 3rd party partner data. Data sources will need to be adapted for real time normalization and correlation.
The pipe then makes its real time data available by an Application Programmable Interface (API) so that any authorized consumer service can read from that pipe in real time. It is up to the consuming service to use, interpret and store the data as required. Traditional ETL processes simply become yet another consumer service to the real time data pipe and from this attachment can use the real time data for big data and any other slow batching analysis that is required.
The pipe enriches the real time data with slow data matching services that make geodemographic and product holding information available to consuming services. The pipe also needs to provide strong, algorithmic, predictive modeling services to the real time data. Ideally this is a real time match to the slow data modeling that occurs in the data warehouse and big data facilities of an enterprise.
Accessible, comprehensible & actionable data
Finally new world analytics systems need to make the real time data accessible, comprehensible & actionable to business users with sandbox style adhoc query and data visualization tools. In doing so they allow a business to scale efficiently beyond the size of a core team of data scientists and technical analysts.
If I had a big data startup with 10 data scientists and it was turning over say $10 million a year in revenue and I went to a venture capital firm seeking growth investment then they would ask, “how many data scientists would you need to turn this into a $100m business or a $1b business”. The answer the venture capital firm wants to hear is 10. It wants to know that the business can scale and that is doesn’t have a data science bottleneck.
Non-technical business users are scalable, data scientists are a rarer commodity. In order to truly benefit from new world data analytics it needs to be safely and scalably made available to business users to access and action. The data engine needs to be placed in the hands of the common business user in order for its true value to be realized.
In any industry, no matter how skilled and novel, there is always the inevitable maturity in process leading to greater accessibility and scalability. The era of new world data analytics is upon us and it is time to evolve or fall behind.
Scott Thomson - Adobe Senior Manager APAC Industry Strategy
Scott Thomson is one of Adobe's APAC industry strategists.
Scott joined Adobe from Telstra where he worked on both the sell side and the buy side of the digital marketing ecosystem as a senior manager in Telstra Media and the Telstra Chief Marketing Office. In his roles at Telstra Scott was involved in digital marketing strategy, transformation, innovation as well as mergers and acquisitions responsibilities.