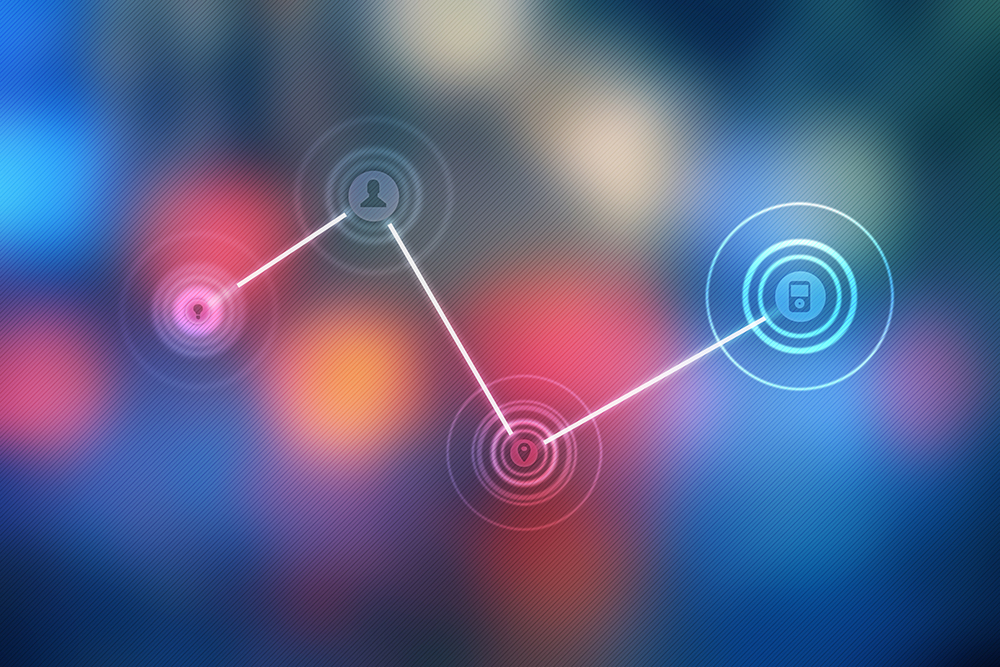
READING TIME: 4 MINUTES
The flux in the C-Suite caused by an acute shift in responsibilities for customer data might be a generalisation, but common sense tells us that as traditional marketing skills become increasingly augmented with sophisticated software capabilities, the impact on business will be far broader than just the brand department.
In every market or industry vertical, one or more businesses are re-calibrating their customer intelligence. From data warehousing, cloud configuration, Extract, Transform, Load (ETL) processes and data visualisation through to segmentation, attribute analysis, campaign management, attribution and CX, the marketing function is flexing its muscles. It’s a high-wire act that can’t shuffle back; the only way is forward.
This tooling up period is complex and is far from risk free. Many RFPs, many pointed questions about someone else’s experience with this or that vendor are a common occurrence. The caution is well-placed – integrations, system compatibilities, unforeseen resource requirements and blatant company politics are predictable hurdles in this MarTech steeple chase.
Yet, when the dust settles, and the logins are confirmed, most enterprises have simply levelled the playing field. Their shiny toy is replicated a hundred times over, across competitors and partners alike. To break the sound barrier, and put distance between itself and its competitors, the enterprise must commit its most ambitious plans to data analysis and exclusive data partnerships.
In short, the MarTech movement isn’t complete without a roll of the dice on the formation of data partnerships involving complimentary and relevant first party data assets.
Determining the Relevancy of a Partnership
The key word here is “relevancy” and is determined by what is sometimes referred to as the conditional distribution of both data sets. This means that there should always be customer attributes in each data set that are of value to the other party, though not necessarily of equal value. An example of two data sets displaying conditional distribution might include an airline and hotel group, or a luxury fashion retail group and premium card provider.
To be sure, the potential data partner is layering new attributes onto an existing customer profile to enhance customer intelligence, but more importantly, the data partnership’s primary objective should be to improve confidence levels in many analytical models relevant to the business, such as forecasting, prediction, propensity and the like. This higher confidence comes from identifying additional attributes in the partner’s data which correlate to improved sales outcomes over multiple time periods.
When there is low conditional distribution between potential data partners (i.e. low relevancy), especially outside the populations of gender and age, there is little value to motivate either party to form a partnership. This common sense test is surprisingly absent in many data-related discussions which are often hijacked by considerations about scale and little more.
The most effective data partnerships encourage each party to learn more about one’s own customers, and to improve the chances of acquiring new, higher yielding customers under existing constraints. The learning process occurs at many levels – from a simple descriptive analysis that might identify a certain proportion of customers are highly engaged sports fans through to a machine learning exercise to reduce customer churn via pre-emptive engagement.
The Rise of Affinity Data in the Partnership Model
Facebook’s Audience Insights and Custom Audiences are examples of a data partnership of sorts, made easier by the near frictionless application of proprietary marketing technology, though still very much confined to Facebook’s walled-garden.
This example of coupling customer identities with interest data obtained by the platform demonstrates how affinity data can be an effective and scalable data partnership option. Admittedly, this particular partnership model is very asymmetrical, undermining its ‘partnership’ credentials, but that doesn’t detract from the power of affinity data playing a key role in customer insights or engagement.
Interestingly, Forrester regards this ‘level’ of affinity data partnership as “simple” and the first of four steps taken in the application of affinity data towards a highly ubiquitous method of data exchange between the savviest of enterprises.
At TEG Analytics, the analysis of affinity data is our mission. Our systems quantify the interests, passions and authentic moments of millions of Australians in the live economy – from the largest football event in the Southern Hemisphere to the most intimate theatre experience; this is affinity data at is most pure and most accountable.
With the evolution of affinity data in mind, the term “smart affinity” is our call sign. TEG Analytics take these data points, with all their transactional and verification qualities, and develops enterprise partnerships to operationalise affinity points via compatible data systems and marketing technologies. Unlike the behemoths with their walled gardens, our smart affinity is symmetrical in philosophy and practice.
The smart affinity approach guides the data partnership to deliver on its promises of audience reach through improved audience design, customer profitability by better understanding the motivations of high yielding customers, and finally enhancing the customer experience via the experiential nature of the data itself.
Transfer Learning: The Key to Data Partnerships
The application of affinity data, also known as the ‘transfer learning’, is central to the value of data partnerships of all persuasions. Enterprises will always insist on maintaining a product or service specialisation, but through the successful application of relevant data partnerships, will learn to identify other customer attributes, not previously captured, which have more of an influence on their customer’s choices than even their own product attributes, marketing or brand equity.
In short, when an enterprise works with reputable data sets which directly enhance product and service experiences, the enterprise is clearly adapting faster than its competitors.
Andrew Reid will be presenting at the 2016 MarTech Symposium.
Andrew Reid
Andrew has more than 18 years experience in digital business management covering the inter-related fields of market research, data management, analytics, digital marketing, product development, programmatic media and marketing automation.